The AI Supercycle represents a fundamental transformation of the global economy, distinct from any technological shift we've seen before.
While we're witnessing rapid changes today, this transformation will mature over 30-50 years, similar to how the microchip revolution reshaped entire industries and job categories across generations.
What makes this cycle unique is its nature as an intelligence layer rather than merely an informational one.
To understand this distinction, consider how the Internet transformed business from the 1990s to the 2020s.
The web acted primarily as an informational layer, starting with outer-layer transformations like distribution channels and gradually moving inward to transform core business models.
The Web vs. AI Integration Pattern
Amazon exemplifies the web integration journey: beginning as an online bookstore (distribution layer), adding digital features (value enhancement), and eventually becoming a comprehensive platform (core transformation).
This outside-in pattern defined the internet era.
In contrast, the AI paradigm works from the inside out. Rather than starting with distribution advantages, AI begins by transforming the core value proposition of products and services.
This fundamental difference means that while distribution was the key battleground of the internet era, in the AI era, superior core capabilities drive success, with distribution advantages emerging as an organic consequence of a 100x value prop.
The AI Stack Architecture
The AI stack reflects this new paradigm through four distinct layers.
At the foundation sits the hardware layer, characterized by massive infrastructure requirements and dominated by a few players like NVIDIA.
Above this lies the foundation models layer, where companies like OpenAI and Anthropic compete to provide core AI capabilities.
The vertical solutions layer follows, offering industry-specific implementations across healthcare, legal, finance, and other sectors.
Finally, the application layer delivers end-user-facing solutions, where both incumbents and startups vie for category leadership.
The Complete AI Stack framework shows how AI integration differs from previous technological paradigms. It consists of four layers:
Hardware: The foundational infrastructure layer
Foundation Models: Core AI capabilities and models
Vertical Solutions: Industry-specific implementations
Applications: End-user-facing products/services
The key insight is that this stack integrates differently than earlier tech waves like the Web.
While web integration started with external layers (distribution) and moved inward to transform core business functions, AI integration starts from the core.
It radiates outward, fundamentally transforming products and services from the inside out.
The Customer Profiles Perspective
The Customer Profile framework outlines three distinct market segments for AI products:
Consumer: High-volume applications with direct user interaction, focusing on ease of use and accessibility. Success depends on user experience and mass adoption.
B2B: Medium-complexity solutions targeting businesses, with emphasis on efficiency and ROI. Requires integration with existing systems and clear value demonstration.
Enterprise: High-complexity, customized solutions with stringent security and compliance requirements. Focuses on scalability, control, and deep system integration.
Each segment demands different go-to-market strategies, product features, and value propositions.
Understanding these distinct profiles is crucial for product development and market positioning in the AI era.
The Distribution Advantage Perspective
The Distribution Advantage framework analyzes how incumbents and startups compete in the AI landscape:
Incumbents start with significant advantages: existing customer relationships, distribution channels, and brand recognition.
However, they face challenges with legacy systems and organizational resistance.
Startups begin with no distribution but can build AI-native solutions without technical debt.
While they struggle with initial market presence and customer acquisition, their clean-slate approach allows for innovative solutions.
The key insight is that unlike the web era, where distribution advantage was critical, in AI, the core product capability can drive distribution.
Strong AI capabilities can naturally lead to market reach, making initial distribution advantages less deterministic of success.
This creates a unique dynamic where incumbents' traditional advantages might be less decisive than previous tech waves.
The Product Integration Levels Perspective
The Product Integration framework identifies three levels of AI implementation:
Basic: Pure LLM integration for fundamental capabilities. This represents the simplest form of AI adoption.
Medium: Combines LLM with RAG (Retrieval Augmented Generation) and search capabilities. This enables more sophisticated contextual understanding and information processing.
Agent: The most advanced level, featuring autonomous AI capabilities. This represents full AI integration with complex decision-making abilities.
The progression through these levels isn't just about technical sophistication—it represents increasing value creation and competitive advantage.
Each level builds upon the previous one, offering greater capabilities and potential for business transformation.
This framework helps organizations understand their current position and plan their AI integration journey.
The Incumbent Paradox
The inherenta developing structure of the AI market might create distinct strategic imperatives for different players.
Incumbents face what might be called the "incumbent paradox" – they possess initial advantages through existing distribution channels and customer relationships but must fundamentally reimagine their core value propositions to survive long-term.
Consider Salesforce's approach: while integrating AI features into their existing platform, they're simultaneously developing AgentForce as a separate, AI-native product line.
Saleforce has hired already thousand of salespeople, which will be trained to sell AgentForce alone.
This dual strategy is critical to enable the current business model to survive in the short term, while long-term success requires complete reinvention.
We’re indeed in the midst of a The Transitional Market Dilemma:
The good news is, as an incumbent is you got time (5-10 years depending on the vertical, as some verticals like EdTech have already been in part disrupted), but, on the other end, the bad news is you got to do it by changing the core.
The 100x Value Prop Snowball Effect
Startups, while lacking established distribution channels, can leverage AI's inside-out nature to their advantage.
By creating products with fundamentally superior value propositions (10-100X improvements), they can achieve rapid distribution as a side effect of their core capabilities.
This dynamic enables a bootstrap-first mentality, where capital follows success rather than preceding it.
That will create a scenario of winner-take-all on the capital employment side, which will make it extremely hard to compete from a venture funding perspective, not that differently from the web era, and yet even more skewed toward a few winners of the capital beauty context!
Incumbents vs. Startups
The Application framework examines how AI integration differs for incumbents versus startups:
Incumbents follow a path of:
Distribution Leg Up: Leveraging existing channels
AI as a Feature: Initial integration
Redefining the Core: Complete transformation
Startups pursue:
10-100X Value Proposition: Focus on massive value creation
Bets and Bootstrap First: Start lean and focused, a very small team can create apps consumed by millions. Of course on the enterprise side, capital will be needed as to develop the enterprise customer is way more expensive, yet the point is with minimum capital you can get quick traction.
Distribution and Capital as Side Effects: Let superior capabilities drive growth
Example: SF approaches through core platform + AgentForce, then standalone products, while startups like Synthesia (Consumer), HeyGen (B2B), and Cohere/Sierra/Writer AI (Enterprise) focus on redefining core value first.
The key insight is that incumbents leverage existing advantages while startups focus on fundamental value creation first.
Investment Strategy in the AI Era
For investors, this paradigm shift demands new deployment strategies.
Capital will be easily available to the top players, and even stronger winner-take-all effects of the web era, will make the run to deploy the capital on the few winners even more skewed.
Visually this is what it looks like.
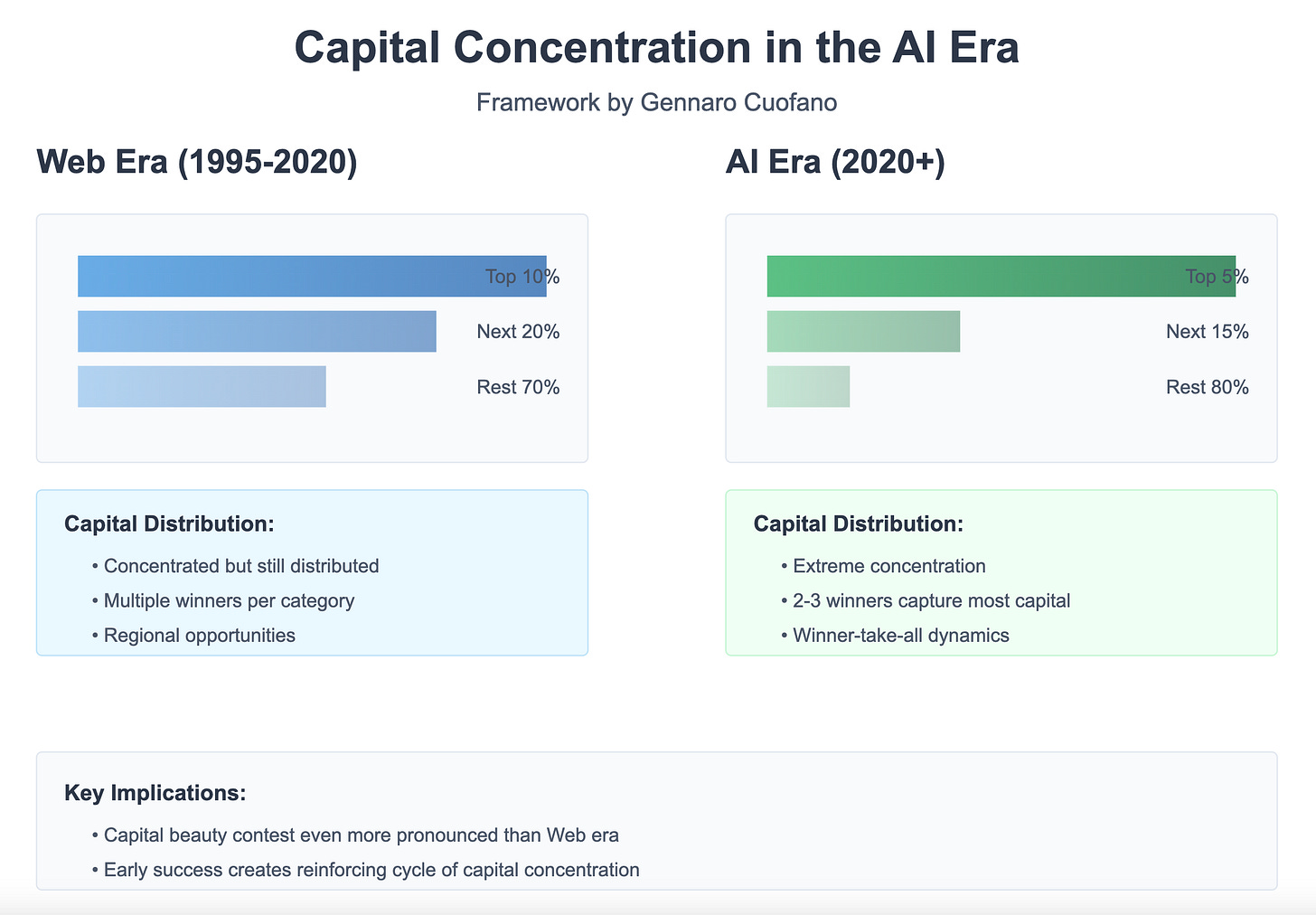
While the hardware and foundation model layers show strong winner-take-all characteristics requiring massive capital, vertical solutions offer more balanced opportunities on the enterprise side, with multiple potential winners per sector.
This creates a barbell strategy where the highest returns might come from either massive infrastructure bets or carefully chosen vertical specialists.
The Capital Deployment framework shows how the "Winner Take All" dynamic varies across the AI stack:
The Foundation Layer shows the highest concentration (2-3 dominant players) due to massive scale advantages and capital requirements.
Hardware Layer and Application Layer also show high concentration but for different reasons - hardware due to infrastructure barriers, applications due to strong network effects, and category dominance.
The Vertical Layer uniquely shows a more moderate distribution, allowing multiple winners per vertical due to industry-specific needs and specialization opportunities.
This understanding is crucial for PE/Family Offices in capital allocation, suggesting different investment strategies based on layer dynamics - concentrated bets in foundation/hardware/applications versus more distributed investments in verticals.
Key implication: While AI drives concentration in most layers, verticals offer more balanced opportunities.
Career Navigation
Perhaps most intriguingly, the AI Supercycle is reshaping career trajectories. Technical professionals can accelerate their growth dramatically, potentially achieving senior positions in 2-3 years rather than the traditional decade-long path. However, this requires a builder's mindset – constantly experimenting with new tools and creating practical applications.
Non-technical professionals must become "specialized generalists," combining broad technological understanding with deep vertical expertise in specific industries.
The Career Navigation framework outlines strategies for thriving in the AI era:
Technical Path: Jump directly to senior build/technical roles, focusing on deep expertise in AI infrastructure and development.
Non-Technical Path: Build networks and develop generalist vertical competencies across multiple industries, emphasizing breadth of understanding over technical depth.
The "Replace Then Create" trajectory of AI suggests that it might first replace existing functions with AI, then create new ones, that we could not imagine could exist.
The key insight is the importance of multiple vertical competencies regardless of path - technical or non-technical professionals both benefit from understanding various industries rather than narrow specialization. This approach differs from previous tech waves by emphasizing broad vertical understanding alongside either technical or network-building expertise.
Long-term Impact On Work
The short-term impact of this transformation may seem alarming, particularly as AI begins to replace certain job functions.
However, just as the microchip eventually created more jobs than it displaced, the AI Supercycle will likely generate entirely new categories of work we cannot yet envision yet.
Recap: In This Issue!
The AI Supercycle Overview
AI represents a 30-50 year transformation, reshaping industries like the microchip revolution.
Distinct from past shifts, AI acts as an intelligence layer rather than an informational one, fundamentally altering products and services.
Web vs. AI Integration Pattern
Web Era: Transformation started with distribution and expanded inward (e.g., Amazon's evolution from bookstore to platform).
AI Era: Transformation starts at the core value proposition and expands outward, prioritizing superior product capabilities over distribution advantages.
The AI Stack Architecture
Hardware Layer: Dominated by players like NVIDIA; requires massive infrastructure.
Foundation Models Layer: Core AI capabilities from companies like OpenAI and Anthropic.
Vertical Solutions Layer: Industry-specific applications (healthcare, finance, legal).
Application Layer: End-user products/services where startups and incumbents compete.
Customer Profiles Perspective
Consumer Segment: Focused on mass adoption and user experience.
B2B Segment: Targets efficiency and ROI with medium complexity.
Enterprise Segment: High-complexity, customized solutions requiring deep integration.
Distribution Advantage Framework
Incumbents: Leverage existing channels but face challenges with legacy systems.
Startups: Lack initial distribution but thrive by building AI-native solutions with superior capabilities.
Product Integration Levels
Basic: Fundamental LLM integration.
Medium: Combines LLMs with advanced tools like RAG.
Agent Level: Autonomous AI capabilities enabling complex decision-making.
The Incumbent Paradox
Incumbents must use their current advantages (distribution, customer base) while reimagining core value to ensure long-term survival.
Example: Salesforce developing AgentForce alongside its platform.
100x Value Proposition for Startups
Startups prioritize creating transformative value (10-100X improvements) to gain market traction, allowing distribution and funding to follow.
Investment Strategy in AI
Foundation Models/Hardware Layers: Winner-take-all dynamics dominate due to high capital requirements.
Vertical Solutions Layer: Allows multiple winners due to diverse, industry-specific needs.
Balanced investment strategies include concentrated bets in foundational layers and distributed investments in verticals.
Career Navigation in the AI Era
Technical Path: Accelerated growth to senior roles by focusing on AI infrastructure and development.
Non-Technical Path: Build networks and gain broad vertical expertise across industries.
With Massive ♥️ Gennaro, The Business Engineer